Measuring Forecast Error
by Phil Stubbs
In the never-ending pursuit of forecasting accuracy, Measuring Forecast Error is an important part of optimising an operation's planning process. Studying the difference between actual values and forecast values can help improve accuracy of future forecasts in a number of ways.
First, there is a great deal to be learned from individual errors. All material errors should be investigated to determine whether there are improvements that can be made to the forecasting model, or whether there was information within the business that might have been useful. A second benefit comes from understanding in the short, medium and longer term, whether the error is increasing or decreasing.
Third, when an analyst is deciding which forecasting model should be deployed, the performance of each candidate model can be tested using a sensible error measure. Following the test, the model that delivers the lowest error can be selected as the one to be used (though this needs to be done with great care to avoid a few regularly-occurring pitfalls).
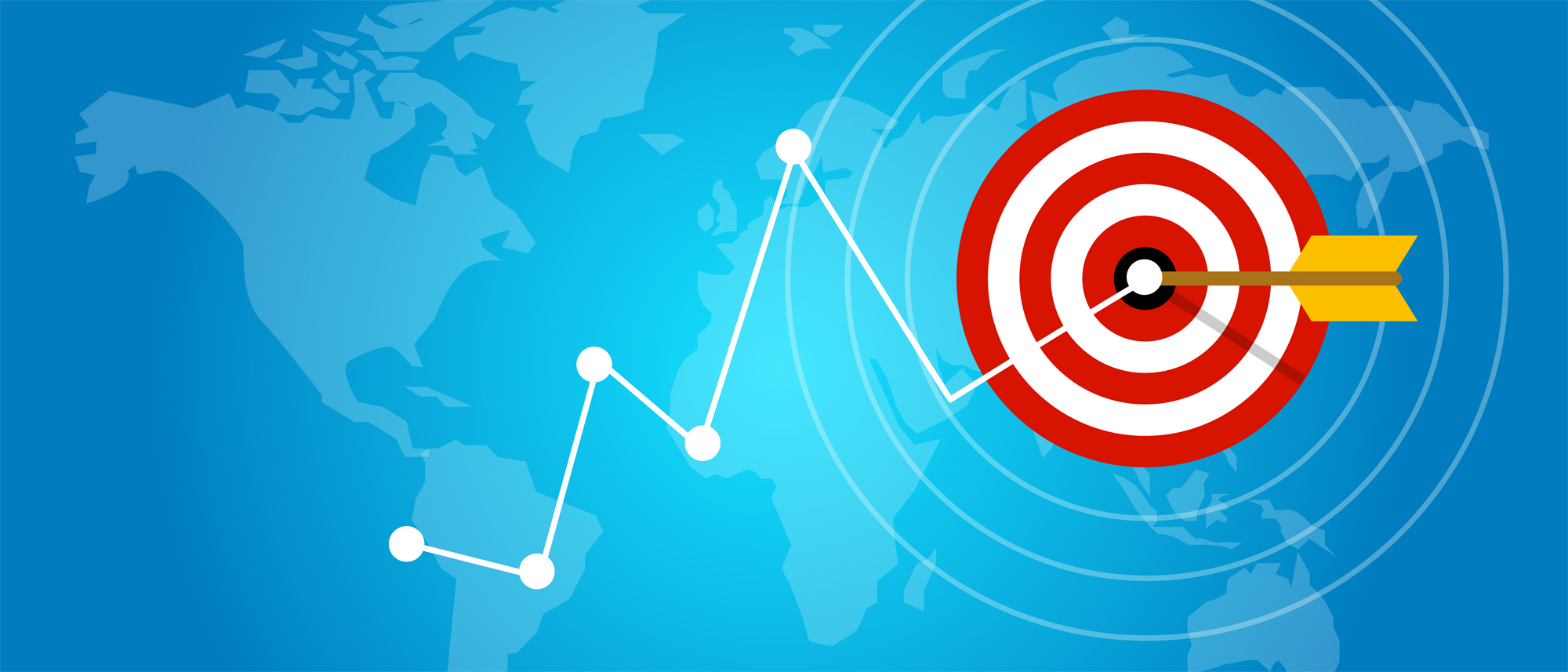
But how should forecast error be measured?
Let’s consider the time series data within the following table, representing two weeks of daily inbound call volume arriving at a fictional Customer Services centre.
Date | Day | Forecast | Actual | Error | Abs Error | % Error | Abs % Err |
Mon | 1 | 26659 | 28316 | 1657 | 1657 | 5.9% | 5.9% |
Tue | 2 | 21581 | 20751 | -830 | 830 | -4.0% | 4.0% |
Wed | 3 | 22867 | 24373 | 1506 | 1506 | 6.2% | 6.2% |
Thu | 4 | 23826 | 23730 | -96 | 96 | -0.4% | 0.4% |
Fri | 5 | 23291 | 22534 | -757 | 757 | -3.4% | 3.4% |
Sat | 6 | 18205 | 18831 | 626 | 626 | 3.3% | 3.3% |
Sun | 7 | 12541 | 11677 | -864 | 864 | -7.4% | 7.4% |
Mon | 8 | 26115 | 27513 | 1398 | 1398 | 5.1% | 5.1% |
Tue | 9 | 18133 | 19748 | 1615 | 1615 | 8.2% | 8.2% |
Wed | 10 | 24656 | 25126 | 470 | 470 | 1.9% | 1.9% |
Thu | 11 | 25968 | 24643 | -1325 | 1325 | -5.4% | 5.4% |
Fri | 12 | 20592 | 22309 | 1717 | 1717 | 7.7% | 7.7% |
Sat | 13 | 19469 | 18294 | -1175 | 1175 | -6.4% | 6.4% |
Sun | 14 | 12339 | 12461 | 122 | 122 | 1.0% | 1.0% |
  |   | ||||||
ME | MAE | MPE | MAPE | ||||
290 | 1011 | 0.87% | 4.72% |
Since Actual = Forecast + Error, we define Error = Actual - Forecast
Across our 14 days, let’s define our first measure:
(1) Mean Error (ME) = Sum of Errors / Number of Forecasts
This measure provides an average of all errors. In our example, the value is 290 calls. The problem we have here is that there are some positive errors and some negative errors, and these can cancel each other out. So this measure can be very small even when there have been large errors. Nevertheless, even with this limitation, this measure remains useful at detecting overall bias in the model, ie whether it tends to overforecast or underforecast over time.
Our second measure deals with that problem:
(2) Mean Absolute Error (MAE) = Sum of Absolute Errors / Number of Forecasts
Here, we make all of the errors positive before we perform the average calculation. In this way, the magnitude of each error is used to calculate the average, irrespective of whether it was positive or negative. In our example, the MAE is 1011 calls, the average magnitude of error.
A disadvantage of measures (1) and (2) is that the results are not comparable across different datasets. Therefore we turn to percentage error, where
Percentage Error = (Actual – Forecast) / Actual
(3) Mean Percentage Error (MPE) = Sum of Percentage Errors / Number of Forecasts
This measure has the limitations of measure (1), but still remains a useful measure of bias, this time expressed as a percentage. Here, the value is 0.87%, which is lower than the absolute value of most of the errors.
(4) Mean Absolute Percentage Error (MAPE) = Sum of Absolute Percentage Errors / Number of Forecasts
MAPE is an extremely useful measure, because it measures the average magnitude of error, as well as providing a method to compare across different forecast variables. Here, the value is 4.72% for our data set. Note that it does not measure bias, so it is to be used in conjunction with measure (3).
Other alternatives measures exist, which may be appropriate to a particular situation, such as Mean Square Error and Root Mean Square Error, which give greater weighting to larger errors. There are even error measurements, such as Theil’s U Statistic, that compare the error from a forecast against a naive method, thus building into the measure the concept of how easy or difficult the time series actually is to forecast. For more information on these, please get in touch.
I hope you found this article useful. Please get in touch with Drakelow Consulting if you have a forecasting or planning problem that you would like further support to resolve. Good luck in improving the accuracy of your forecasts!