
Drakelow Consulting's Forecasting Tips 1-5
by Philip Stubbs
Here are the first five of the Forecasting tips that I posted on LinkedIn earlier this year...
Tip 1 - Get to know your data intimately; ensure it is accurate
This is critically important for accurate forecasting. Never take anything for granted about your data. It is worth investing substantial time to understand the data you are exploiting to make forecasts. In all likelihood, this will mean getting to know the data tables and the queries used to derive the data.
First, carefully study the historical data of the variable you aim to forecast, to ensure it is robust and that it reports what you think it does.
There are many examples of checks to ensure the data is robust. If you are forecasting sales volume, make sure that you are capturing all of the relevant sales in your historical data, and that all of the sales are actually taking place within the specified time period.
If you are forecasting email arrival volumes, make sure the data you are using reports number of emails arriving and not those handled. If you are forecasting call volume in order to estimate advisor requirement, make sure that you are reporting all of the different call types that are making their way to the advisors.
Second, the same rule also applies to historical data of the explanatory variables. If there are problems with this data, it will make it harder to find relationships to explain variation in what you are trying to forecast. In particular, make sure that the explanatory variable data has the same definition of time period as the forecast variable.
Tip 2 - Understand the concepts of Trend and Seasonality
I often encounter a lack of clarity amongst professionals when discussing the terms Trend and Seasonality, so today’s tip is to ensure you grasp these two concepts well. In the charts below are examples of Trend and Seasonality.
Once you have grasped these terms, it is much easier to discuss variation in historical data, which we will do in the next tip.
By the way, a bugbear of mine is when people use the term “seasonal trend”. To me, it doesn’t really mean anything. Stick to the separate terms of seasonality and trend when you describe data.
A Trend is a long term increase or decrease in the demand data. The chart below, with 28 data points, shows a positive trend.

The chart below - again with 28 data points - shows a negative trend.

The time series data in the graph below has no trend.

Seasonality is a regularly repeating pattern with identical duration. This daily data has a seasonality of 7.

The chart below contains monthly sales data over five years. This data has seasonality and no trend. This monthly data has a seasonality of 12.

This quarterly data below has seasonality AND a positive trend. It has a seasonality of 4.

Tip 3 - Fully understand the causes of historical variation
Before you start forecasting a particular demand line, take time to understand the variation within the historical data.
I would recommend plotting a chart: I find that I notice much variation in a chart that I would miss looking at a column of numbers. The relevant period of historical data will vary, depending on its availability and usefulness. Maybe label the chart with the variation explanations.
Ask questions such as: How much of the variation in the data is attributable to seasonality and trend? Have there been periods of unusually high or low volume that can be explained by a factor, such as the announcement of a price change? Has something happened during the time period to cause a long term sustained increase or decrease in the data, for example, the introduction of new technology? How much can be explained away by other factors?
If you cannot answer these questions yourself, ask anyone who might know the answer.
You may find that it is difficult to pinpoint reasons for variation if you don’t have the information. For this reason, I recommend all forecasters keep a digital diary of the causes of unusual variation, so that variation knowledge is not forgotten.
Tip 4 - Explore the relationship between two variables
If you think that some historical variation is due to the impact of an independent variable, then a scatterplot can help provide more understanding. It shows whether there is a mutual relationship between two variables. A straight-line relationship is known as correlation.
With a scatterplot, it’s the statistical convention to plot the independent variable on the x-axis (left to right along the bottom) and what you are trying to forecast on the y-axis (top to bottom on the left).
In the first example below, we see sales volume along the x-axis and inbound call volume on the y-axis.
A scatterplot is easy to create in Excel and other statistical packages.
A correlation in itself provides no proof of cause-and-effect, it just shows that there is a straight-line relationship between the two variables.
Try and find relationships between variables within your data.
In the chart below, each data point represents one week. The data demonstrates a positive correlation. As number of sales increases, so does the number of inbound calls – with a broadly straight line.
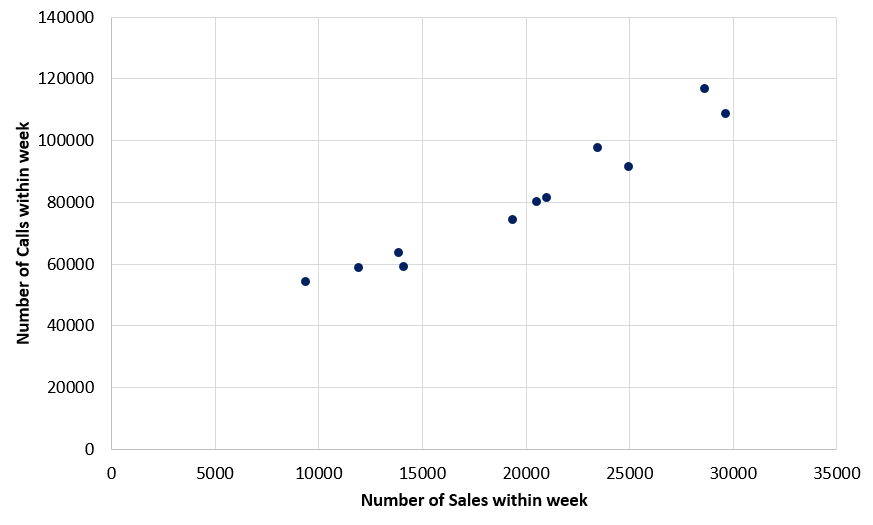
The data below demonstrates Perfect Positive Correlation. A correlation where all of the points are exactly in a straight line is called perfect.
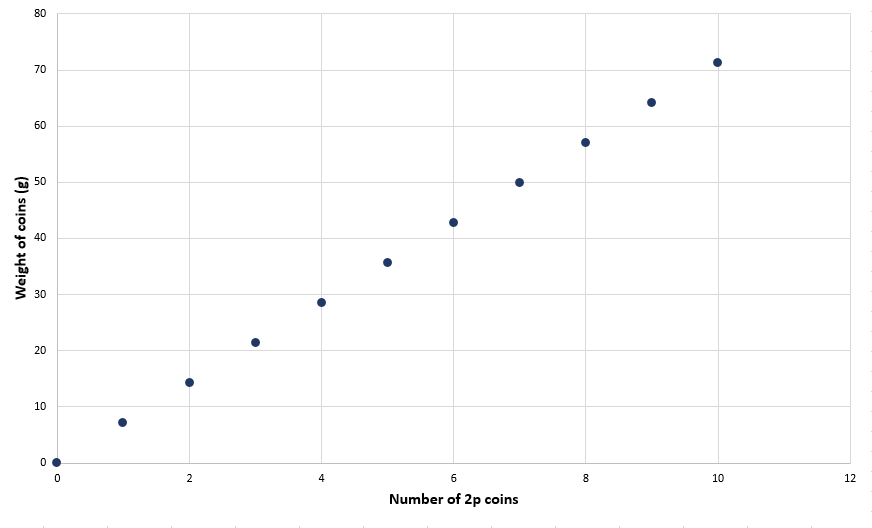
The data below demonstrates Negative Correlation. As one variable increases, then the other variable decrease.
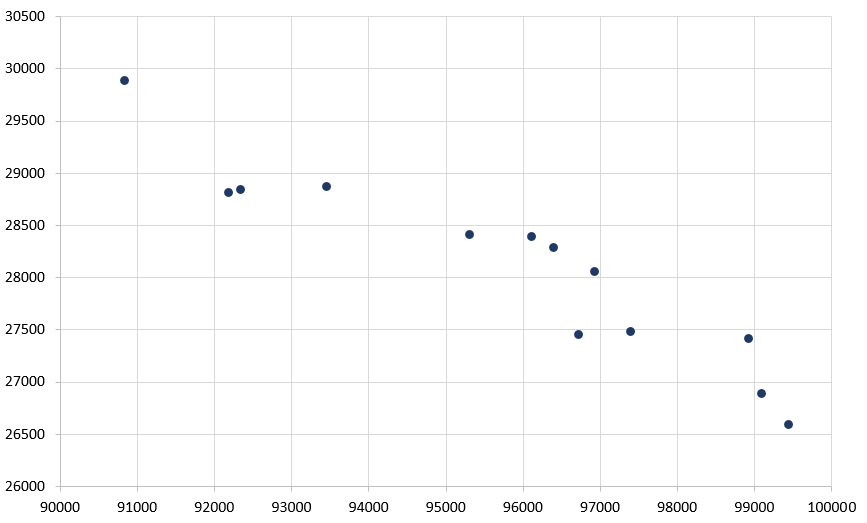
There is no correlation when there is no linear relationship.

Tip 5 - Make the forecast as late as you can
When you are responsible for producing a regular forecast, for example each week, a key decision is when is the best time to perform the forecast?
When negotiating forecast delivery times, bear in mind that the later the forecast is made, the more information you will have to take into account. Such information includes recent historical data and ever-changing promotion plans.
For example, if a forecast is required by the user every Wednesday at 1pm, then don’t finalise the forecast on a Monday. Instead, if you are able to complete the forecast on the Wednesday morning then do so, because you will have two more days of historical data, and you may have more up-to-date information about an external factor that you didn’t know about on the Monday.
When you are agreeing delivery times for forecasts, try and make them as late as possible, while still providing enough time to the user to take action with the forecasting insight. By taking the most up-to-date data into account, this will help maximise the accuracy of the forecast, and therefore its usefulness.
More catch-up tips will be published here soon.
Drakelow Consulting offers full support to business wishing to improve their forecasting and planning capability, including improving data, models and processes. We can also provide tailored coaching and training to ensure your analysis teams have the skills and experience required to deliver the accuracy you desire.
If you would like to discuss how to improve the accuracy and usefulness of your forecasting, across any or all of these fundamentals, then please contact us at hello@drakelowconsulting.com